Since joining our Multi-Asset Team earlier this year as a fixed income analyst, Sally Hoang has really enjoyed the cross-asset lens that’s used when analysing investments. Combined with her background as a pharmacist, it helps her understand the potential for AI in healthcare.
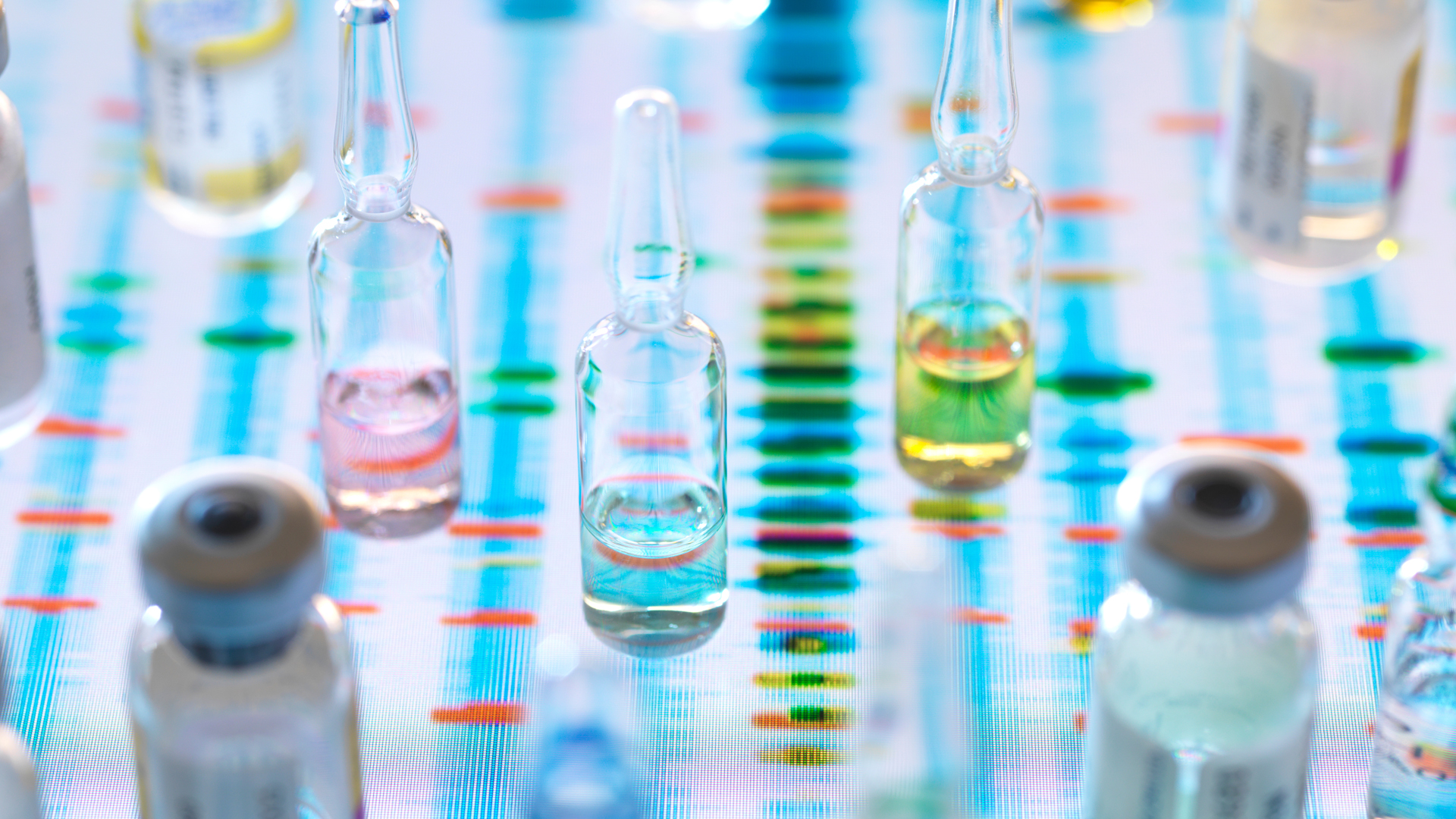
AI: just what the doctor ordered
In a past life, many years ago, I was a pharmacist working at a public hospital. I saw patients every day, assisting them with all things medicines-related.
During my pharmacist training, I remember spending a whole summer as part of a ‘scholarship’ (which I am partly convinced was just an excuse for cheaper labour) working in a science laboratory. As the radio played that summer’s hottest hits on repeat, I struggled to breathe the thick air of the science lab through my mask and safety goggles. I was tasked with helping a research associate with experiments on optimising the synergy of antibiotic combinations against bacterial ‘superbugs’. In basic terms, that involved me swabbing different concentrations of a Pseudomonas Aeruginosa, a virulent bacteria, on to petri dishes with different concentrations and combinations of antibiotics, incubating to grow the bacteria, and then counting how many colonies of bacteria grew (often well over 100). Fun. I still wonder whether my summer could have been better spent. I’ll never know.
At the time, I remember a big driver for the research was rising antibiotic resistance and the lack of new antibiotics to fight infections. Did I think we were going to discover the next penicillin to tackle infections? Not really. But a part of me couldn’t help but draw parallels with the discovery of Penicillin by Alexander Fleming in 1928 where he essentially discovered by complete accident that a Penicillium mould, which he later named Penicillin, had antibacterial effects. This became a hugely important scientific breakthrough. I’m sure most of us have used Penicillin at some point or another to treat an infection. At bottom, science is often trial and error – it’s throwing combinations together and monitoring what happens. And this is where I think artificial intelligence (AI) could really strap rockets to pharmaceutical development.
it’s already starting
As AI improves, more and more uses are coming into frame. Lots of companies across many different sectors are working to integrate AI into their operations and workflows to improve efficiencies. Yet I can’t help but shake the idea that AI could save a great many lab technicians countless hours of monotonous work.
Gone are the days where academics spent hours trawling through academic literature to try to better understand data. And gone are the days where new drugs are discovered through trial and error or ‘by accident’. Or, at least, the trials can be better targeted by AI programs determining from past data what is most likely to work. Think faster discovery of drugs that are better designed to meet their therapeutic targets. Think accelerated clinical trials that no longer take years or even decades of time and money. Think (hopefully) faster approval by regulators such as the Food and Drug Administration (FDA) in the US.
In the last few years pharmaceutical companies have really leaned into AI, with just about every large-cap pharma company running some kind of partnership or project involving AI. Uses have ranged across the whole drug discovery process and include finding targets for drugs, designing drugs, clinical trials and manufacturing. One such drug discovered through AI was Pfizer’s PAXLOVID, an oral COVID-19 treatment approved in 2022, demonstrating the power of AI in drug discovery. Pfizer used modelling and simulation to screen millions of compounds and then virtually selected the right molecular structure that would ensure that potency was enhanced. Supercomputing also performed complex calculations to assist in the drug being available in oral form rather than as an injectable.
We hold Swiss pharma Roche across our Multi-Asset Portfolio funds, which has a number of AI-related initiatives under way. One of them, Genentech, has a strategy centred on creating a ‘lab in a loop’ where data is fed into AI models and algorithms to assist in identifying trends and predictions, as well as identifying new drug molecules. Scientists then perform experiments based on the outcomes, and the information is again fed through the model, which further improves the drug, forming an iterative cycle or ‘the loop’. This has helped Genentech enhance its efforts in discovering exciting treatments such as personalised cancer vaccines which are still in the works. Roche and Genentech are collaborating with tech companies, like Nvidia (which we also own), to introduce generative AI to further enhance discovery and development of new drugs.
Roche has adopted AI in other parts of its business as well. In 2021, using AI and cloud technologies in partnership with Microsoft (another business in our portfolios), Roche developed a breast cancer diagnosis tool with an accuracy of 96%. In the past, mammograms (X-rays used to look for cancers in breasts), were usually interpreted by a number of people– the first being the provider of the primary diagnosis, the second usually to confirm, and in the case of complex or debatable cases, a third person was also involved. The technology works as a first ‘screener’. In positive cases, this screening indicates where the abnormal cells are located and assigns an abnormality score. This information is then reviewed and confirmed (or not) by a skilled radiologist. The whole process is made more efficient and more accurate with the help of AI.
A part of me still wonders what I would have done that summer I spent in the science lab if AI had been around. Would I still have needed to swab bacteria on to petri dishes and count the colonies? Or would AI have been able to come up with a solution? Or, at the very least, count the hundreds and thousands of bacterial colonies for me!
Tune in to The Sharpe End - a multi-asset investing podcast from Rathbones. You can listen here or whenever you get your podcasts. New episodes monthly.